Four Types Of Hand Plane 75,Wood Whittling Kit 011,Fine Woodworking Lathe Review Js,Best Carpenter Tools Zoom - Step 3

In a drilling machine, we use bevel gear to transmit power at an angle of 90 degrees. The power transmission in the drilling machine used to transmit power for its working. The process of transmission takes place with the help of the v-bolt and the pair of pulley stacks opposite to each other. In the market there are various types of Drilling machine available, here I mention some of the popular types of drilling machine s.
The sensitive drilling machine has only a hand-feed mechanism for feeding the tool into the workpiece. This enables the operator to feel how the drill is cutting and accordingly he can control the down feed pressure. Vertical or Pillar Drilling Machine is free standing and is of a far heavier construction able to take larger drills.
The larger drills normally have a taper shank located within taper bore in the spindle end. These tapers are standardized as Morse tapers. The radial drill machine is free-standing and the workpiece is clamped in the position on the base.
It is used for heavy large and heavy work. The arm is power-driven for the height location. The drill head is positioned using motorized drives and it transverse the swinging arm.
In the Multi-spindle drilling machine, there are many spindles mounted on one head to allow many holes to be drilled simultaneously.
N umerical control drilling machine can automatically change tooling with a turret or automatic tool changer. It is designed with cones like internal structure, narrow at the top of the web with a gradually increasing thickness to the shank. It is a multi-point cutting tool. I also wrote an article on the single-point cutting tool you can check that too.
The portion of the drill extending from the sank or next to the outer corners of the cutting lips. The angle included between the chisel angle and the cutting lips as viewed Types Of Hand Plane Tools Unity from the end of the drill. Helical or Street grooves cut or formed in the body of the drill to provide cutting lips, to permit removal of chips and to allow cutting Fluids to reach the cutting lips. The length from the outer corners of the cutting lips to the extreme back and of the flutes; it includes the sweep of the tool used to generate the flutes and, therefore does not indicate the usable length of the flutes.
The distance between the leading edge and the hill of the land measured at the right angle to the leading edge. The axial relief angle at the outer corner of the lip; it is measured by projection onto a plane tangent to the Periphery at the outer corner of the lip. The length from the extreme end of the shank to the outer corners of the cutting lips; it does not include the conical shank end often used on a straight shank drill, nor does it include the conical cutting point used on both straight and taper shank drills.
The cutting end of the drill made up of the end of the lands and the web; inform it resembles a cone, but departs from a true cone to furnish clearance behind the cutting lips.
The angle included between the cutting lips projected upon a plane parallel to the drill axis and parallel to the two cutting lips. The central portion of the body that joins the land; the extreme end of the web forms the chisel edge is on a two-flute drill.
These are the following operations that can be performed in the Drilling machine. When we need a circular hole in a workpiece of any size there, we can use drilling operation, by a drilling operation you can form any size of holes in a workpiece.
Although you can use a lathe for drilling operation too, drill machine is an appropriate machine to do holes in a workpiece. The cutting tool we used for this type of operation is drill bit. A drill bit is a multipoint rotary cutting tool which helps to remove material from a workpiece. When sand castings are made, cores are used to displace the metal where holes are desired. When cast the molten metal flows around the core. After the metal solidifies the casting is removed from the mold and the core disintegrates leaving the desired holes.
When you need to enlarge the diameter of the existing hole you need to perform the boring operation , but the accuracy is not greater than reaming operation. The boring tool is generally a single-point cutting tool. A finished hole has the specified diameter size, is perfectly round, the diameter is the same size from end to end, and it has a smoothly finished surface.
A drill hole is seldom accurate enough in size or sufficiently smooth to be called a precision hole. When greater accuracy is required the whole must be drilled undersize by a certain amount and finished by the reaming. In short, When we need to enlarge the size of an existing hole with great accuracy in a workpiece we have to performed reaming operation. In this type of operation, we need a reamer to perform the operation.
It is one of the first neural networks to demonstrate learning of latent variables hidden units. Boltzmann machine learning was at first slow to simulate, but the contrastive divergence algorithm speeds up training for Boltzmann machines and Products of Experts. The self-organizing map SOM uses unsupervised learning. A set of neurons learn to map points in an input space to coordinates in an output space. The input space can have different dimensions and topology from the output space, and SOM attempts to preserve these.
Learning vector quantization LVQ can be interpreted as a neural network architecture. Prototypical representatives of the classes parameterize, together with an appropriate distance measure, in a distance-based classification scheme. Simple recurrent networks have three layers, with the addition of a set of "context units" in the input layer. These units connect from the hidden layer or the output layer with a fixed weight of one. The fixed back connections leave a copy of the previous values of the hidden units in the context units since they propagate over the connections before the learning rule is applied.
Reservoir computing is a computation framework that may be viewed as an extension of neural networks. A readout mechanism is trained to map the reservoir to the desired output. Training is performed only at the readout stage.
Liquid-state machines [57] are two major types of reservoir computing. The echo state network ESN employs a sparsely connected random hidden layer. The weights of output neurons are the only part of the network that are trained.
ESN are good at reproducing certain time series. The long short-term memory LSTM [54] avoids the vanishing gradient problem. It works even when with long delays between inputs and can handle signals that mix Four Types Of Hand Plane University low and high frequency components.
Bi-directional RNN, or BRNN, use a finite sequence to predict or label each element of a sequence based on both the past and future context of the element. The combined outputs are the predictions of the teacher-given target signals. This technique proved to be especially useful when combined with LSTM. Hierarchical RNN connects elements in various ways to decompose hierarchical behavior into useful subprograms. A stochastic neural network introduces random variations into the network.
Such random variations can be viewed as a form of statistical sampling , such as Monte Carlo sampling. A RNN often a LSTM where a series is decomposed into a number of scales where every scale informs the primary length between two consecutive points.
A first order scale consists of a normal RNN, a second order consists of all points separated by two indices and so on. The Nth order RNN connects the first and last node. The outputs from all the Different Types Of Hand Planers Journal various scales are treated as a Committee of Machines and the associated scores are used genetically for the next iteration.
Biological studies have shown that the human brain operates as a collection of small networks. This realization gave birth to the concept of modular neural networks , in which several small networks cooperate or compete to solve problems. A committee of machines CoM is a collection of different neural networks that together "vote" on a given example.
This generally gives a much better result than individual networks. Because neural networks suffer from local minima, starting with the same architecture and training but using randomly different initial weights often gives vastly different results.
The CoM is similar to the general machine learning bagging method, except that the necessary variety of machines in the committee is obtained by training from different starting weights rather than training on different randomly selected subsets of the training data. The associative neural network ASNN is an extension of committee of machines that combines multiple feedforward neural networks and the k-nearest neighbor technique.
It uses the correlation between ensemble responses as a measure of distance amid the analyzed cases for the kNN.
This corrects the Bias of the neural network ensemble. An associative neural network has a memory that can coincide with the training set. If new data become available, the network instantly improves its predictive ability and provides data approximation self-learns without retraining.
Another important feature of ASNN is the possibility to interpret neural network results by analysis of correlations between data cases in the space of models. A physical neural network includes electrically adjustable resistance material to simulate artificial synapses. Instantaneously trained neural networks ITNN were inspired by the phenomenon of short-term learning that seems to occur instantaneously.
In these networks the weights of the hidden and the output layers are mapped directly from the training vector data. Ordinarily, they work on binary data, but versions for continuous data that require small additional processing exist. Spiking neural networks SNN explicitly consider the timing of inputs. The network input and output are usually represented as a series of spikes delta function or more complex shapes.
SNN can process information in the time domain signals that vary over time. They are often implemented as recurrent networks. SNN are also a form of pulse computer. Spiking neural networks with axonal conduction delays exhibit polychronization, and hence could have a very large memory capacity. A regulatory feedback network makes inferences using negative feedback. It is most similar to a non-parametric method but is different from K-nearest neighbor in that it mathematically emulates feedforward networks.
The neocognitron is a hierarchical, multilayered network that was modeled after the visual cortex. It uses multiple types of units, originally two, called simple and complex cells , as a cascading model for use in pattern recognition tasks. Local features in the input are integrated gradually and classified at higher layers.
Compound hierarchical-deep models compose deep networks with non-parametric Bayesian models. However, these architectures are poor at learning novel classes with few examples, because all network units are involved in representing the input a distributed representation and must be adjusted together high degree of freedom.
Limiting the degree of freedom reduces the number of parameters to learn, facilitating learning of new classes from few examples. Hierarchical Bayesian HB models allow learning from few examples, for example [89] [90] [91] [92] [93] for computer vision, statistics and cognitive science.
Compound HD architectures aim to integrate characteristics of both HB and deep networks. It is a full generative model , generalized from abstract concepts flowing through the model layers, which is able to synthesize new examples in novel classes that look "reasonably" natural. All the levels are learned jointly by maximizing a joint log-probability score.
A deep predictive coding network DPCN is a predictive coding scheme that uses top-down information to empirically adjust the priors needed for a bottom-up inference procedure by means of a deep, locally connected, generative model. This works by extracting sparse features from time-varying observations using a linear dynamical model. Then, a pooling strategy is used to learn invariant feature representations.
These units compose to form a deep architecture and are trained by greedy layer-wise unsupervised learning. The layers constitute a kind of Markov chain such that the states at any layer depend only on the preceding and succeeding layers. DPCNs predict the representation of the layer, by using a top-down approach using the information in upper layer and temporal dependencies from previous states.
DPCNs can be extended to form a convolutional network. Multilayer kernel machines MKM are a way of learning highly nonlinear functions by iterative application of weakly nonlinear kernels.
They use kernel principal component analysis KPCA , [96] as a method for the unsupervised greedy layer-wise pre-training step of deep learning. To reduce the dimensionaliity of the updated representation in each layer, a supervised strategy selects the best informative features among features extracted by KPCA.
The process is:. A more straightforward way to use kernel machines for deep learning was developed for spoken language understanding. The number of levels in the deep convex network is a hyper-parameter of the overall system, to be determined by cross validation. Dynamic neural networks address nonlinear multivariate behaviour and include learning of time-dependent behaviour, such as transient phenomena and delay effects.
Techniques to estimate a system process from observed data fall under the general category of system identification. Cascade correlation is an architecture and supervised learning algorithm. Instead of just adjusting the weights in a network of fixed topology, [99] Cascade-Correlation begins with a minimal network, then automatically trains and adds new hidden units one by one, creating a multi-layer structure. Once a new hidden unit has been added to the network, its input-side weights are frozen.
This unit then becomes a permanent feature-detector in the network, available for producing outputs or for creating other, more complex feature detectors. The Cascade-Correlation architecture has several advantages: It learns quickly, determines its own size and topology, retains the structures it has built even if the training set changes and requires no backpropagation.
A neuro-fuzzy network is a fuzzy inference system in the body of an artificial neural network. Depending on the FIS type, several layers simulate the processes involved in a fuzzy inference-like fuzzification, inference, aggregation and defuzzification. Compositional pattern-producing networks CPPNs are a variation of artificial neural networks which differ in their set of activation functions and how they are applied.
While typical artificial neural networks often contain only sigmoid functions and sometimes Gaussian functions , CPPNs can include both types of functions and many others. Furthermore, unlike typical artificial neural networks, CPPNs Different Types Of Hand Planes Queen are applied across the entire space of possible inputs so that they can represent a complete image. Since they are compositions of functions, CPPNs in effect encode images at infinite resolution and can be sampled for a particular display at whatever resolution is optimal.
Memory networks [] [] incorporate long-term memory. The long-term memory can be read and written to, with the goal of using it for prediction. These models have been applied in the context of question answering QA where the long-term memory effectively acts as a dynamic knowledge base and the output is a textual response. In sparse distributed memory or hierarchical temporal memory , the patterns encoded by neural networks are used as addresses for content-addressable memory , with "neurons" essentially serving as address encoders and decoders.
However, the early controllers of such memories were not differentiable. This type of network can add new patterns without re-training. It is done by creating a specific memory structure, which assigns each new pattern to an orthogonal plane using adjacently connected hierarchical arrays.
Hierarchical temporal memory HTM models some of the structural and algorithmic properties of the neocortex. HTM is a biomimetic model based on memory-prediction theory.
HTM is a method for discovering and inferring the high-level causes of observed input patterns and sequences, thus building an increasingly complex model of the world. HTM combines existing ideas to mimic the neocortex with a simple design that provides many capabilities. HTM combines and extends approaches used in Bayesian networks , spatial and temporal clustering algorithms, while using a tree-shaped hierarchy of nodes that is common in neural networks. Holographic Associative Memory HAM is an analog, correlation-based, associative, stimulus-response system.
Information is mapped onto the phase orientation of complex numbers. The memory is effective for associative memory tasks, generalization and pattern recognition with changeable attention. Dynamic search localization is central to biological memory. In visual perception, humans focus on specific objects in a pattern. Humans can change focus from object to object without learning.
HAM can mimic this ability by creating explicit representations for focus. It uses a bi-modal representation of pattern and a hologram-like complex spherical weight state-space. HAMs are useful for optical realization because the underlying hyper-spherical computations can be implemented with optical computation. Apart from long short-term memory LSTM , other approaches also added differentiable memory to recurrent functions. For example:. Neural Turing machines [] couple LSTM networks to external memory resources, with which they can interact by attentional processes.
The combined system is analogous to a Turing machine but is differentiable end-to-end, allowing it to be efficiently trained by gradient descent. Preliminary results demonstrate that neural Turing machines can infer simple algorithms such as copying, sorting and associative recall from input and output examples. They out-performed Neural turing machines, long short-term memory systems and memory networks on sequence-processing tasks.
Approaches that represent previous experiences directly and use a similar experience to form a local model are often called nearest neighbour or k-nearest neighbors methods. Documents similar to a query document can then be found by accessing all the addresses that differ by only a few bits from the address of the query document. Unlike sparse distributed memory that operates on bit addresses, semantic hashing works on 32 or bit addresses found in a conventional computer architecture.
Deep neural networks can be potentially improved by deepening and parameter reduction, while maintaining trainability. While training extremely deep e. Such systems operate on probability distribution vectors stored in memory cells and registers. Thus, the model is fully differentiable and trains end-to-end. The key characteristic of these models is that their depth, the size of their short-term memory, and the number of parameters can be altered independently.
Encoder—decoder frameworks are based on neural networks that map highly structured input to highly structured output. The approach arose in the context of machine translation , [] [] [] where the input and output are written sentences in two natural languages.
Access best practices and settings to deliver secure tests in your course with WebAssign. Learn More. Have you used the same assignments and questions each semester? Explore new content types available for your course that address top teaching challenges. Try Something New. Get the most out of your course in WebAssign with live and recorded webinars that provide peers tips and best practices. Explore More.
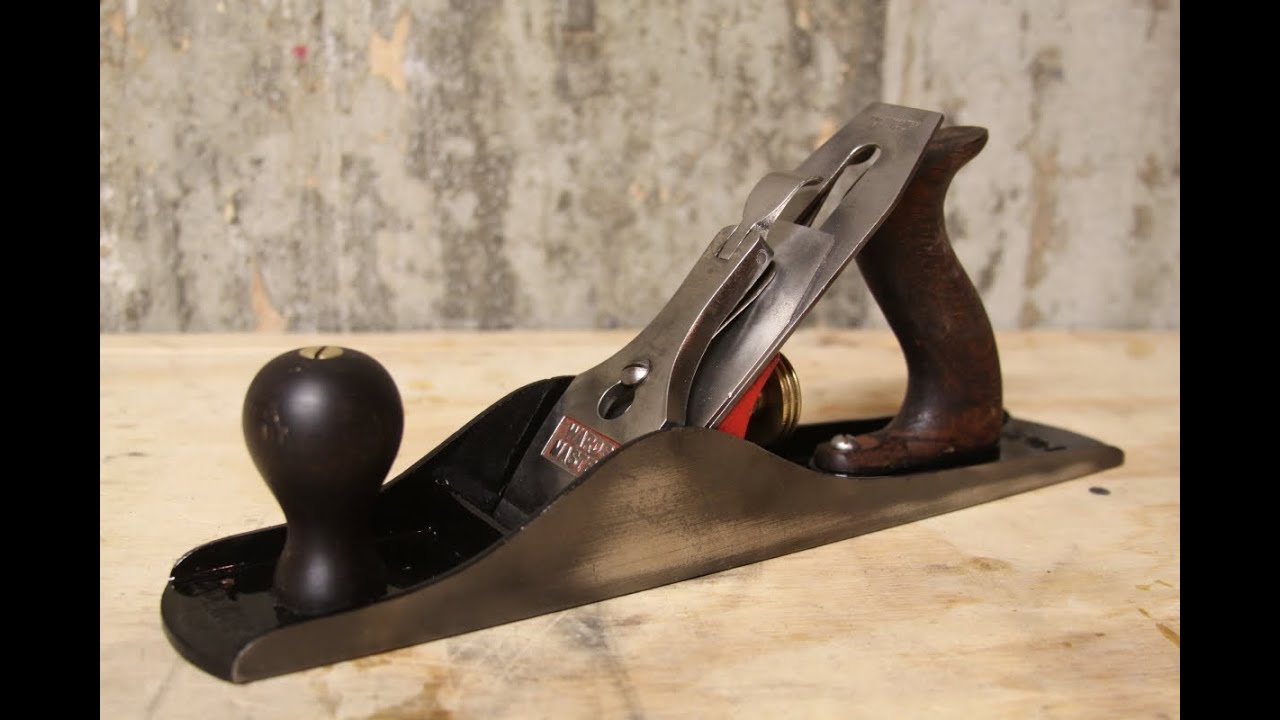


Lumber Products Airline Quotes Best Rap Songs Early 2000s |
KPACOTKA
21.10.2020 at 20:58:59
ASKA_SURGUN
21.10.2020 at 18:44:20
KRUTOY_BMW
21.10.2020 at 21:54:57
horoshaya
21.10.2020 at 16:39:19