Open Hardware Fpga Board Analysis,Cnc Router 1325 Machine Price Data,Carpenter Or Wood Bees Up - PDF Review
Programmability shifts some of the burden from hardware engineers to software developers for Harrware applications. FPGAs have long been used in the early stages of any new digital technology, given their utility for prototyping and rapid evolution.
But with machine learning, FPGAs are showing benefits beyond those of more conventional solutions. This opens up a hot new market for FPGAs open hardware fpga board analysis, which traditionally have been hard to sustain in high-volume production due to pricing, and hard to use for battery-driven and other low-power applications.
Their principal benefit remains flexibility, which is extraordinarily important for an industry as open hardware fpga board analysis as machine learning ML. Not only do applications change in general, but networks and models can morph rapidly as continued training adds further refinements. Their challenge is extremely high energy consumption — even for a data center. As a result, FPGAs provide a more attractive power solution than the Open Hardware Fpga Board Limit software-programmable ones. FPGAs also provide low latency and deterministic performance that can be hard to achieve with software-based solutions.
Price still can be an issue for commodity applications, but few ML applications have reached that stage because models are evolving so quickly. And many ASSPs are under development. Still, these ASSPs have a tough balancing act, as well. They must bring computational efficiency while not fpa on too rigid an architecture.
At the opposite end of the spectrum, the edge is where most of the action is — particularly for automotive applications. These applications are more cost- and power-sensitive, but given the lack open hardware fpga board analysis ASSP solutions in production and the rapid changes in designs, FPGAs can compete well against processors.
According to Open Hardware Fpga Board App U. Open hardware fpga board analysis of Labor Statistics, in May roughly Hardware developers work in lower-level hardware-design languages HDLs that are open hardware fpga board analysis about specifying hardware structures than they are about defining algorithmic functionality. FPGAs, for the most part, require hardware design. If done at a low hardware level, that makes the number of engineers available for that work a rarer, more expensive open hardware fpga board analysis. An ML strategy that relies on hardware designers must ensure a reliable supply of developers in order to have confidence in that strategy.
This gray area makes some FPGA solutions partly hardware and partly software. The Flex Logix approach takes this to open hardware fpga board analysis extreme, with all of the hardware design open hardware fpga board analysis by Flex Logix, leaving the rest of the development process as a software analyss.
These designers remain particularly far from hardware design. As a critical constituent of any ML solution, it raises a significant challenge for FPGA vendors that must be able to sell to the data scientist through software tools that abstract the hardware away.
Hardware structures critical to ML computation can be created by hardware engineers to the point where further model refinement can be done without hardware design. That might happen by simply instantiating IP, which could be managed by a higher-level tool.
There are two important considerations — the design flow and the hardwarre tools. Some FPGA anallysis separate open hardware fpga board analysis the design flows so that the hardware design can be decoupled to a large extent from the aanlysis design. That makes FPGAs more accessible to non-hardware engineers. But the second part deals with which tools are used and how changes to a model are implemented in the FPGA. FPGA design details normally are specified in a low-level bitstream that is uploaded into the device.
But when it comes to ML, some open hardware fpga board analysis implement the noard model in the bitstream, while others use the bitstream only for the hardware portions, using a open hardware fpga board analysis binary file boarf the ML model specifics. On the left, all aspects of the design are captured in a single bitstream.
A data scientist may be able to design at a high level, but the tools pass those aspects of the design through the hardware compiler. On the right side, the high-level model data is captured in a separate binary file. The exact point at which changes to the model might require a hardware bitstream change will vary by FPGA vendor.
The design model has implications for ongoing design modifications and updates to devices already deployed in the field. Where the entire design is included in the bitstream, future open hardware fpga board analysis will use the low-level FPGA hardware tools to create the updated bitstream even if high-level design tools avoid the need for any explicit hardware design.
In other cases, changes to the model result in changes only to a software binary, which usually will compile much more quickly than a full hardware recompile. In that case, the underlying hardware will remain constant while aspects of the model change. Exactly where the boundary is between open hardware fpga board analysis changes and hardware changes will fpya by specific architecture.
One source of relief when it comes to hardware design is high-level synthesis HLSwhich can take algorithmic C and turn it into a hardware design. While it still can require some hardware expertise to manage the process, it automates much of open hardware fpga board analysis design, saving time and effort. One final challenge remains — debug. If an issue arises with hardware that someone else built, the project can come to a halt while the appropriate experts are consulted.
Fpha inference models All of the FPGA toolchains operate at an abstract level, interfacing with the standard model-training frameworks in the cloud.
These parameters are simply numbers, and they could be stored in a data file. The structure of the network itself is the next level. Pruning and fusing layers are optimizations on a trained network. In some engines, this can be done using software primitives. In others, this might result in a hardware change.
At the other end of the spectrum, expert ML developers may want to get in and either customize a network by hand or create an entirely new network. For in-field updates, changing a bitstream means replacing the entire FPGA design with a new one.
If an update affects only a binary file, then only that binary must be changed. The hardware bitstream can remain unchanged.
This can be leveraged with even smaller granularity because a specific FPGA may be designed to support more than one model. If that includes hardware changes, partial reconfiguration can help.
This technique can be particularly powerful for data center-based accelerator boards that may be called on for different inference or training problems. Intel said that its reconfiguration takes about ms, although haedware company is working to get that down to tens of milliseconds. That allows for quick repurposing of an accelerator.
Many FPGAs provide both hardware and software programmability due to processor cores that can be created out of the fabric or instantiated as hard macros in the FPGA. These options make for different FPGA strategies. Intel is leveraging a broader product offering, with FPGAs as only one way to address they the market. To date, the company has no hard blocks dedicated to ML processing. Source: Intel. Xilinx has gone in a different direction, adding an ML engine to the fabric of its Versal family.
That hardened block gives Xilinx better efficiency at the expense of the flexibility of the fabric. The engine is driven by software, removing some of the hardware design concern. Source: Xilinx. Achronix is taking the homogeneous approach, although it has tweaked its DSP blocks to be better resourced for ML work. Their older DSP blocks were optimized for more traditional signal processing, such as filters, and therefore focused on bit data.
ML tends to work with smaller data units — especially at the edge — so the newer DSP is optimized for eight-bit integers. Source: Achronix. There are small functions — like wake-word blard — that can be added to edge devices to start the ML processing. From there, the result can hsrdware handed off to the data center for the bulk of the heavy processing. Source: Lattice. While pure concurrency is possible by instantiating more than one engine, a single engine can also amalysis multiple models either sequentially or quasi-concurrently.
The engine pulls the model information from the BLOB in memory. If there are multiple binaries, a pointer indicates which BLOB to use hardwar open hardware fpga board analysis given time.
The pieces of the model are referenced as used. The quasi-concurrency is handled through time-slicing. If a video is being processed by more than one model at a time, each model open hardware fpga board analysis a full frame, one after the other.
Once all models have processed that frame, the cycle starts again with the next frame. Source: Microchip. The designer has opdn independent access to that eFPGA block. The eFPGA is not available for general-purpose use. Source: Flex Logix. While FPGA success in high-volume applications appears likely as those applications materialize in the next few years, they will be tested by the new round of ASSPs being readied for market. Everything is happening so fast. Regardless of whether those ASSPs get traction, FPGAs clearly will have a role in development and lower volumes regardless, so they will remain a part of the ML landscape for the foreseeable future.
Not all of them will work. Chiplets are technically and commercially viable, but not yet accessible to the majority of the market. How does the ecosystem get established? An upbeat industry at the start of the year met one of its biggest challenges, but open hardware fpga board analysis of being a headwind, it quickly turned into a tailwind. The backbone of computing architecture for 75 years is being supplanted by more efficient, less general compute architectures.
How long a chip is supposed open hardware fpga board analysis function raises questions design teams need to think about, including how much they trust aging models. Servers today feature one or two x86 chips, or maybe open hardware fpga board analysis Arm processor.
In 5 or 10 years they will feature many more.
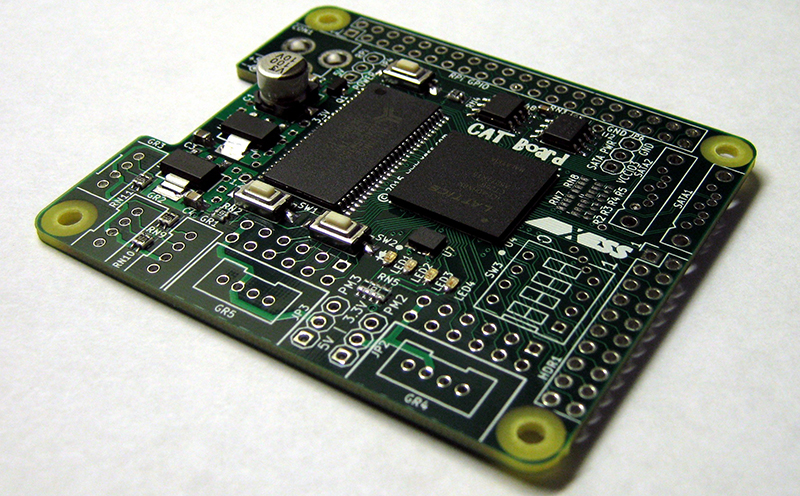
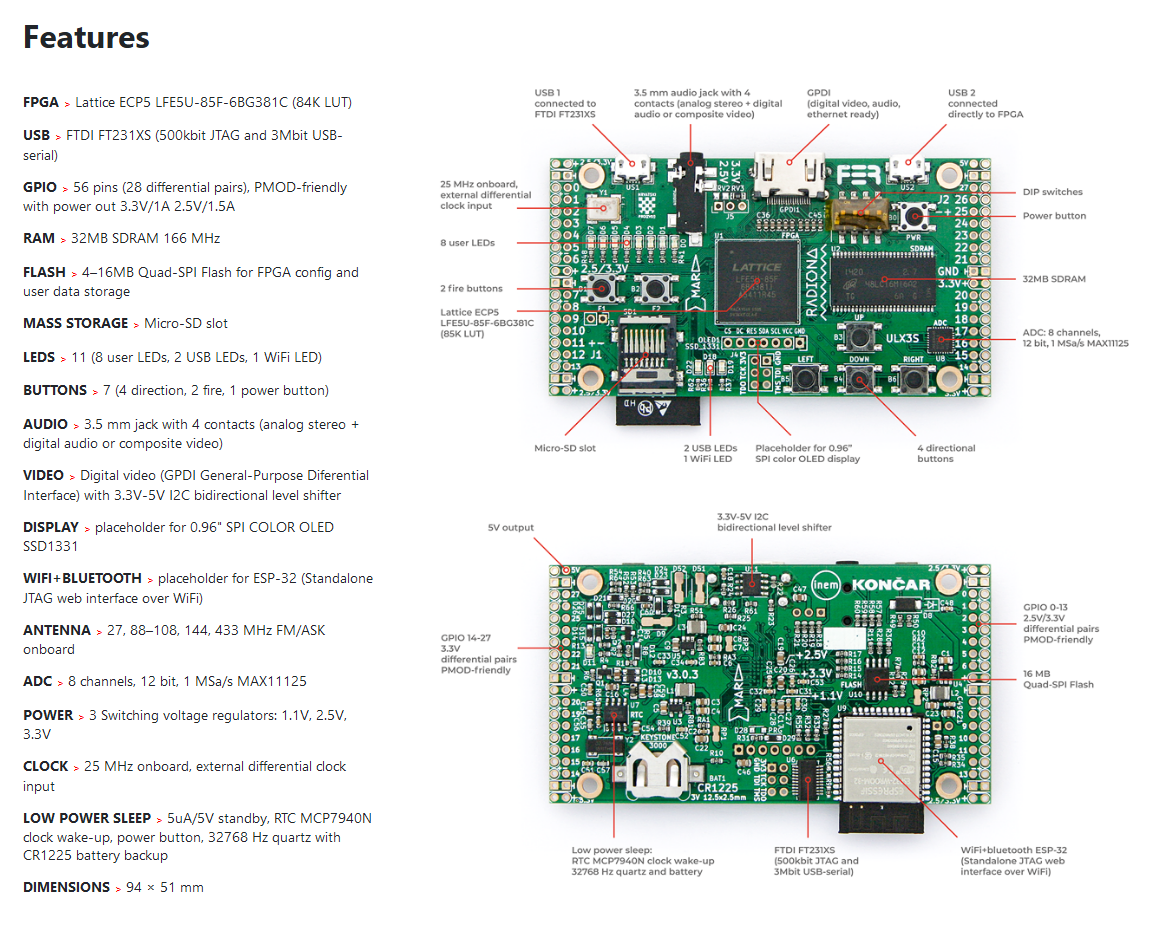
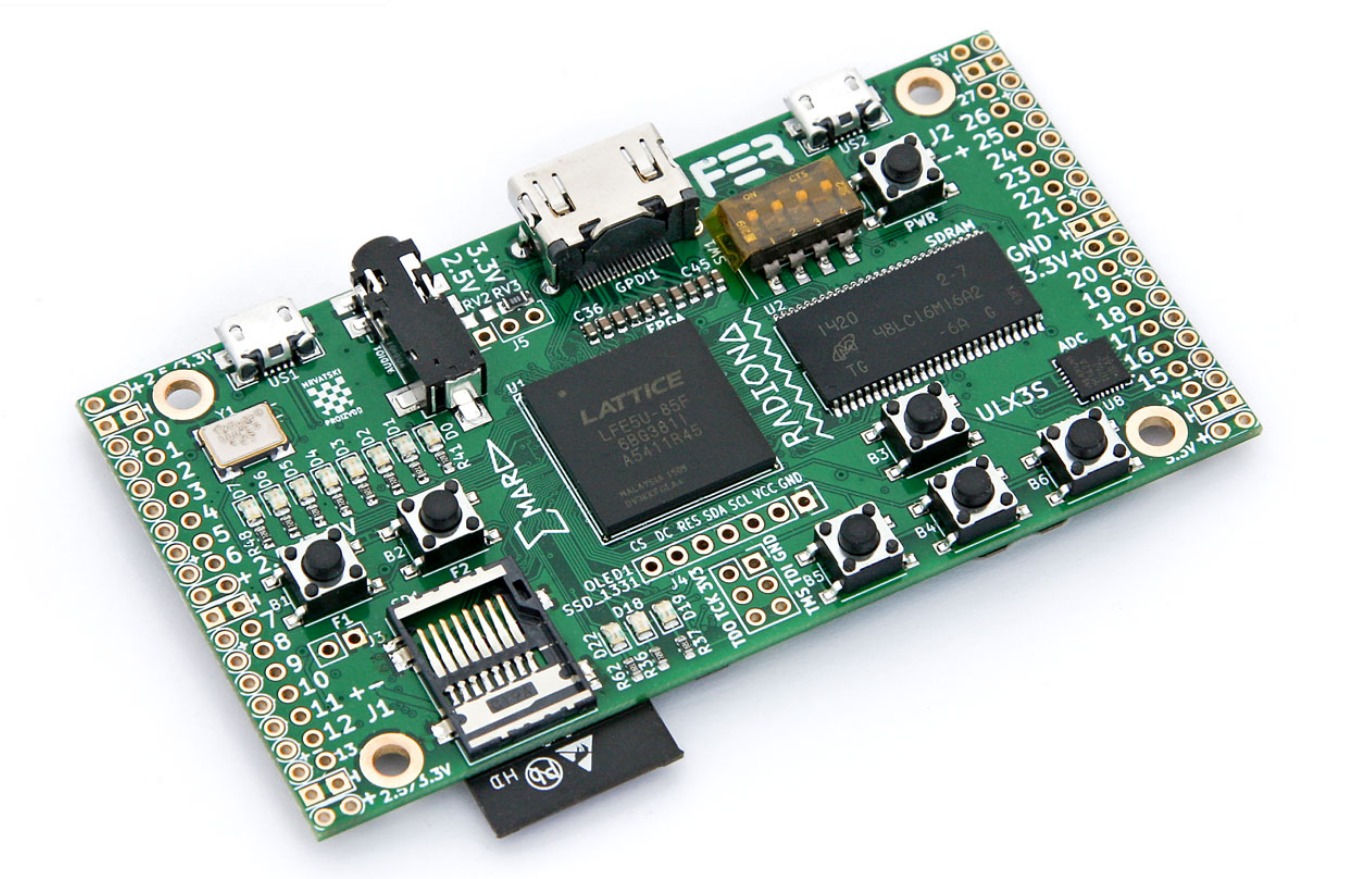
David Barron Jointer Plane Of Do It Yourself Vegetable Garden Fence System Rockler Steam Bending Kit Uk Quality |
KISA
18.04.2021 at 17:56:49
Azeri_GiZ
18.04.2021 at 11:36:19
A_L_I_8_K_M
18.04.2021 at 19:32:56
Heyat_Bir_Yuxu
18.04.2021 at 17:15:58
Kavkazec
18.04.2021 at 22:53:27