Lumber Products Can Be Modeled Best Using The Model Of Quantitative,Router Bits For Baseboard Trim Number,Indoor Crafts For Adults Quit,Router Table Build Id - Good Point

Our Programs. Log In. Contact Us. Real Estate Development Projects Aggregates. Energy We're committed to clean, renewable options that meet environmental standards and coexist with sustainable forestry. Sustainability We're focused on climate change solutions, creating sustainable homes for everyone, and supporting thriving rural communities.
CEO Message. Investors For more than a hundred years, Weyerhaeuser has been building and enhancing a reputation for responsible corporate citizenship. Careers We offer a range of exciting career opportunities for smart, talented people who are passionate about making a difference.
Forests in the Southern U. Forests in the Northeastern U. Forests in Canada Log Sales U. South Log Sales U. Your preferred supplier for more than years. Western Lumber Our eight sawmills in the western U. Southern Lumber With a full offering of visual Lumber Products Can Be Modeled Best Using The Model Of Korea and machine stress rated products, our Southern Pine is an outstanding choice for framing and treated wood applications.
Take a step up with our lumber products and service offerings: Innovative products and services designed to meet your unique needs Unrivaled manufacturing capability, reliably producing quality lumber products World class production forecasting, logistics support and supply chain management expertise.
Request a Quote Contact us for a quote on lumber products. The revenue per log was obtained by multiplying the Lumber Products Can Be Modeled Best Using The Model Of Cell amount of board footage of each grade by its corresponding market value. Table 3. In addition to developing a model to predict the log yields and revenue using the Monte Carlo method, an MLR model was implemented with the goal of comparing both methods with the real lumber yields.
According to the results in the literature, the length, diameter, and log grade can be used as predictors for the log yields. Therefore, the data from the same 24 log groups used to simulate the log yields with the Monte Carlo method were used to estimate the coefficients of the MLR models. The coefficients to predict the board footage by grade are shown in Table 5 and statistical output is presented in Appendix in Table A1 to Table A3.
For each log group diameter, clear face, and length , the corresponding intercept and coefficients were used to estimate the board footage by grade. The revenue per log was calculated using the grade market prices, as in previous calculations. The predicted board footage for each log group and revenue is displayed in Table 6.
This MLR model had a decent fit as the R 2 value observed was 0. This low P-value and moderate R 2 value indicates that the deviations in the predictors were correlated to alterations in the response variable.
Thus this MLR model explained substantial response variability based on variation in input parameter. Also, the adjusted R 2 , the proportion of total variance that is explained by MLR model, was 0.
With three predictors and adjusted R 2 value of 0. Additionally, the root mean square error of the whole model was To estimate the yield with the MLR model, multicollinearity between variables can be the major limiting factor for precise outputs. To estimate the precise yield from MLR equation, all the variables in a regression model should behave independently. Diameter has the highest VIF of 3. This observation indicated that all three variables were moderately correlated as per the rule of the thumb described by Ott and Longnecker As VIF for this prediction model is considerably lower than 5, multicollinearity will not be the major limitation to predict the lumber yield.
As the VIF of the variables is moderately correlated, it also fulfills the assumption of Monte Carlo simulation as independent variables. This suggested that when the log quality was higher and the diameter and length were larger, a more accurate prediction of the log yield was obtained using the Monte Carlo method.
Table 7. The prediction results from the MLR by log group were less precise than the results obtained from the Monte Carlo method Table 7. This suggested that the MLR had less accuracy than the Monte Carlo method when the log quality and dimensions were smaller. In summary, when both prediction models were compared against the total average real log yield revenue, it was found that the MLR model had an overall error of 3.
This indicated that as an aggregate, both models performed well, but the resulting data suggested that it was better to look at each individual log group rather than the aggregate data.
Finally, Fig. This research provides an important step to assist hardwood sawmills in developing better models to estimate lumber yields based on specific log characteristics. Log grading systems are designed with the goal of separating or classifying logs by the potential gross revenue based on the lumber grade yields.
A common agreement among lumber producers and academics is that lumber grade yields are highly variable, but there are some fundamental characteristics, such as the scaling diameter, length, and log grade, that highly correlate with lumber yields.
However, the difficult task of predicting log grade yields from the intrinsic yield variability is still one of the main drivers for the industry to estimate the gross revenue or profitability over an extended period of time and large log batches, rather than focusing on the potential gross revenue from individual logs or smaller log batches. Historically, hardwood sawmills have not been enthusiastic about adopting new information from previous lumber yield studies due to a lack of resources and the complexity.
Nevertheless, with the advancement of information technologies, data analytics have taken a step forward to develop quicker, more effective, and simple data prediction models that can support sawmills in making better decisions to quickly react to changes in pricing or a lack of raw material. In this study, a model was developed to estimate lumber grade yields based on the Monte Carlo simulation. This method does not force the data to a specific deterministic equation, but rather fits the data to a probability distribution that can explain variability better than deterministic models.
By hypothesizing that the behavior of each lumber grade yield is different, the implementation of the Monte Carlo method revealed that lumber grade Lumber Products Can Be Modeled Best Using The Model Of Work yields behave differently for each lumber grade. In this study, input analysis was applied to a set of 24 log groups each with 10 different lumber grades, which resulted in a variety of fitted PDFs.
The need to use a variety of distribution functions showed that lumber grade yields are associated with many different variables that are difficult to separate and analyze on their own. This research demonstrated that the major advantage of the Monte Carlo simulation was the ability to precisely predict the output of each log group individually. In real applications, knowledge of the possible volume and gross revenue of specific log groups can be beneficial for production planning, inventory control, and log purchasing.
Mayer and Wiedenbeck reported that sawmills have not had access to data analytics to make such decisions for individual logs or smaller batches, but this model can be broken down into individual logs or log groups. Another major advantage is the capability to adapt to a sudden change in the input parameters in the final results, which would make it easier for a hardwood sawmill to use this model to instantly predict lumber grade yields and gross revenue from available logs within the inventory.
Additionally, the prediction can be improved continuously by adjusting the ICPDF parameters after incorporating additional log groups and more data on the lumber grade yields. In contrast to the Monte Carlo method, the MLR model predicted lumber grade yields and gross revenue as a single point estimator.
For lumber grade yields, the MLR model had a better prediction accuracy than other linear regression models, but the major problem with this type of estimation was the limitation of the model in incorporating variability.
It was found in this research that estimated MLR equations were unable to explain the basic relationship of the variables and outcomes. These results suggested that the MLR increased its prediction accuracy only when the value of the variables diameter, clear face, and length were closer to their mean values. The results also indicated that an estimation of the lumber grade yields based on the grade depended on the dimensions and quality of the log.
Differences in the gross revenue of different log qualities and dimensions were evident. This finding was similar to that of other research, which has concluded that the most important factors of the yield recovery are the diameter, length, log grade, milling methods, and forest site conditions.
Though not all of these variables were used in this research, the findings still reinforced what has been reported in previous literature. Additionally, this study only included log groups with sample sizes equal to or greater than Thus, it is necessary to include additional log groups with higher sample sizes in future research if possible.
Finally, the results of this study could be used by practitioners to estimate with high confidence the potential revenue from a specific set of logs or log inventory that matches the log groups analyzed in this study. An analytical tool can be easily developed in Excel that can perform the simulation for a particular log inventory. The authors are very grateful to Dr.
Curt Hassler and the Appalachian Hardwood Center at West Virginia University for the temporary loan of their proprietary log and lumber yield data, allowing us to conduct this research. Agatonovic-Kustrin, S. DOI: Auty, D. Forest Res. Billinton, R. Buehlmann, U. Clark, S. Cumbo, D. Denig, J.
Grushecky, S. Hogg, R. JMP- statistical software- Kretschmann, D. Luppold, W. Mayer, R. Offered By. University of Pennsylvania. About this Course How can you put data to work for you? Career direction. Career Benefit. Shareable Certificate. Flexible deadlines. Hours to complete. Available languages. Instructor rating. Offered by. Week 1. Video 7 videos. Reading 1 reading. PDF of Lecture Slides 10m.
Quiz 1 practice exercise. Module 1: Introduction to Models Quiz 30m. Week 2. Video 6 videos. Module 2: Linear Models and Optimization Quiz 30m. Week 3. Video 12 videos. Module 3: Probabilistic Models Quiz 30m. Week 4. Video 8 videos.
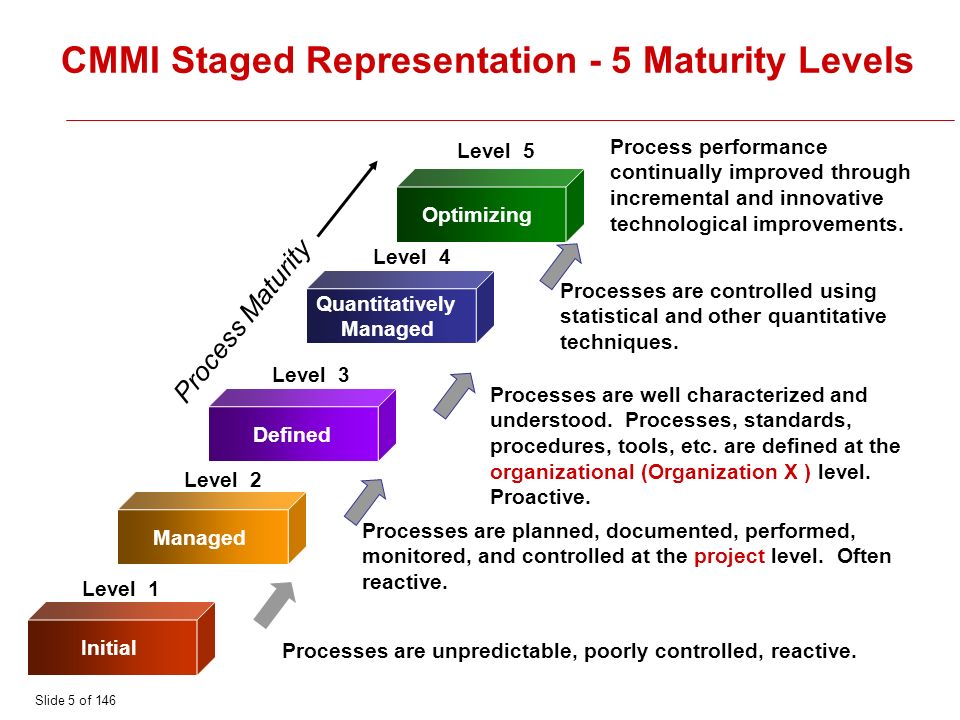
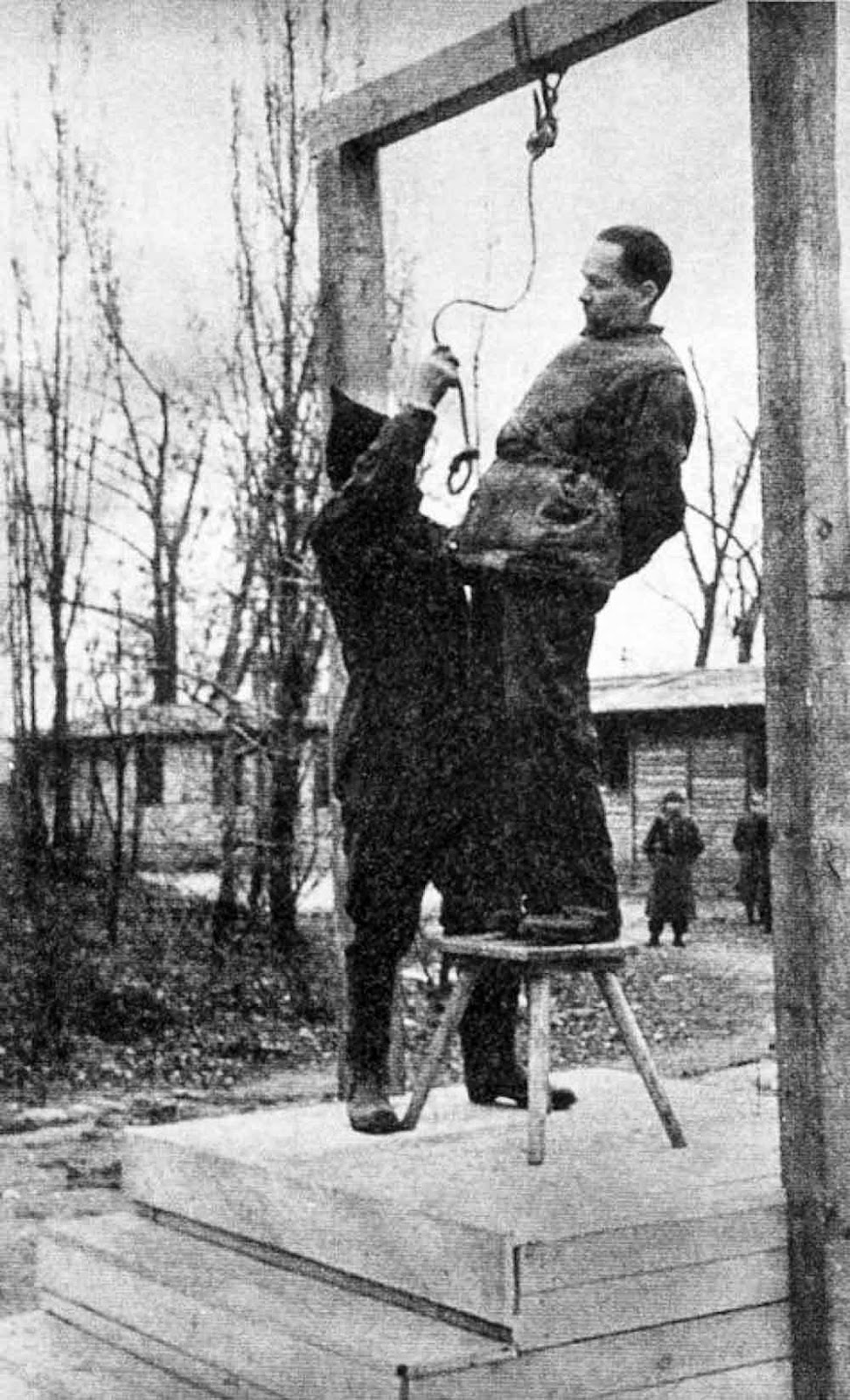.jpg)
Blum 21 Inch Drawer Slides Ltd Best Wood For Turning Bowls Uk University Top Selling Woodworking Items List Picture Framing Tools And Supplies Usa |
ERDAL_23
25.07.2021 at 20:50:15
Natcist
25.07.2021 at 12:42:45
bakililar
25.07.2021 at 15:51:10
ANTIKVAR
25.07.2021 at 22:25:39